Five Johns Hopkins Scientists Among 83 Who Will Share in $15 Million Award From Chan Zuckerberg Initiative to Fund Computer-Based Research on Human Cells
04/19/2018
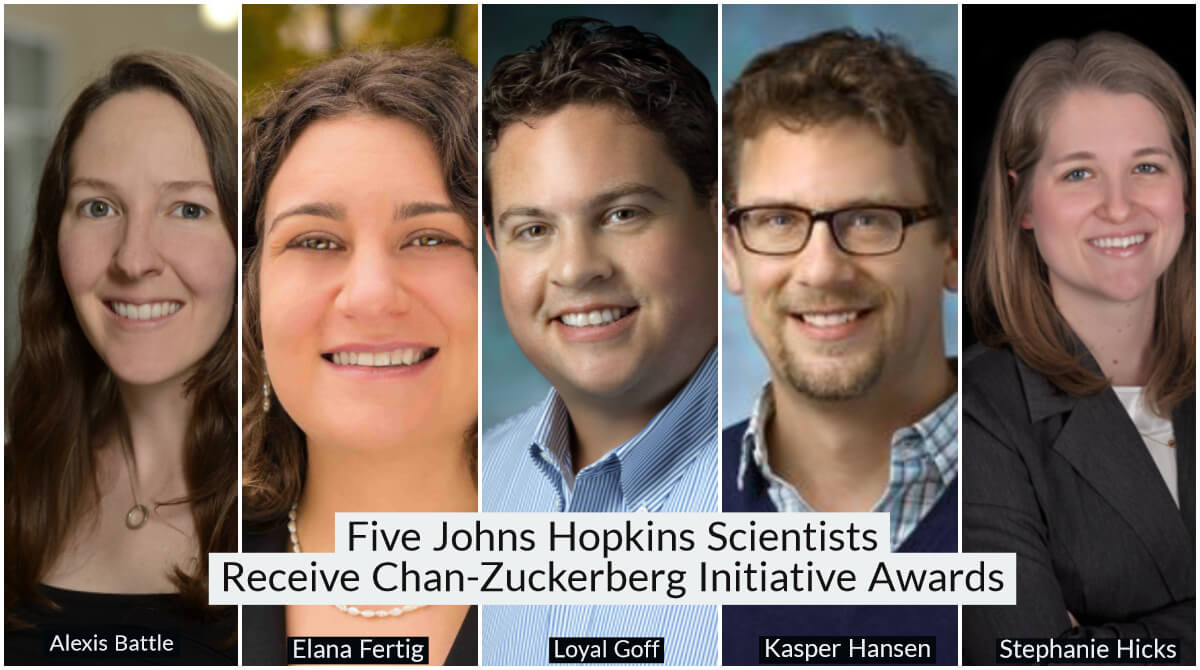
The award, which is called Collaborative Computational Tools for the Human Cell Atlas, has been given to scientists who will develop open access tools that use computing, algorithms and other ways to visualize, collect and analyze molecular and imaging data being generated by scientists working on the Human Cell Atlas. The atlas is an effort to create an encyclopedia of molecular and imaging data that charts every cell in the human body.
“We are excited to begin working on these promising projects with new partners from across the globe. These grantees include experts in experimental biology, engineering and computational biology. Enabling them to collaborate and bring their diverse perspectives to the work is the core of our approach to advancing biomedical science,” said Cori Bargmann, head of science for the Chan Zuckerberg Initiative.
The one-year grants are provided by the Chan Zuckerberg Initiative DAF, an advised fund of Silicon Valley Community Foundation. Scientists are required to share their data online and with the larger consortium of researchers involved in the Human Cell Atlas. Facebook founder Mark Zuckerberg and his wife Priscilla Chan founded the Chan Zuckerberg Initiative in 2015. It is a philanthropic organization that supports basic biomedical research, education and social justice issues.
The five awardees from Johns Hopkins are working on projects that include:
Finding the Elusive Altered Cell Among Millions of Normal Ones
Scientist Alexis Battle will be using probabilistic models to analyze the output of genes in single cells from multiple individuals in order to understand the differences between genetic effects on different cells. Differences between cells within a certain tissue may not be apparent when all the cells in a tissue are measured together, since most human tissues are a mix of multiple different cell types. Finding these changes among multiple people can help explain disease processes. Providing a way for scientists to pinpoint these changes in a specific cell type within a tissue and across larger populations of people can improve our understanding and detection of disease.
Alexis Battle, Ph.D.
Assistant Professor of Biomedical Engineering
Johns Hopkins University Whiting School of Engineering
Lessons Learned from Human Cells
Mathematical algorithms that detect patterns may reveal how healthy tissues change over time. Scientist Elana Fertig will be combining her experience in developing weather prediction systems for the NASA Goddard Space Center and University of Maryland with her cancer genomics research at The Johns Hopkins University to analyze data from human cells. She will be looking for molecular changes that occur during tissue development and how cells process information during the development of more complex systems, such as the eye. Understanding how healthy cells change over time can provide researchers with clues to what happens when information goes haywire and normal cells develop into cancer.
Elana Fertig, Ph.D.
Assistant Professor of Oncology
Assistant Director, Quantitative Sciences, Division of Biostatistics and Bioinformatics
Johns Hopkins Kimmel Cancer Center
Teaching Computers to Learn How the Retina Is Formed
Experts in computational and single cell biology will be teaching computers to “learn” how individual stem cells in the retina turn genes on and off at different times to form different cell types. To do this, they will be using machine-learning techniques to examine the relationships and patterns between human and mouse retinal cells. Their newly developed computer software adapts a method called “transfer learning,” which uses information learned from one set of data and transfers that knowledge to analyze new data. The benchmark and training data they create for retinal cells may be useful for datasets across the entire Human Cell Atlas.
Loyal Goff, Ph.D.
Assistant Professor of Neuroscience
Johns Hopkins University School of Medicine
McKusick-Nathans Institute of Genetic Medicine
Open Source Software That Can Analyze Millions or Billions of Single Cells
You have more cells than you can imagine. Now you have to analyze all of them. How do researchers do this? Researchers Kasper Hansen and Stephanie Hicks are building on an open source software project called Bioconductor for statistical analysis and visualization of genomic data. Hansen and Hicks, in concert with other Bioconductor developers, will extend this software to build the infrastructure and tools needed to analyze tens of millions, or even billions, of a variety of single cells.
Kasper Hansen, Ph.D.
Assistant Professor of Biostatistics
Johns Hopkins Bloomberg School of Public Health
Stephanie Hicks, Ph.D.
Assistant Professor of Biostatistics
Johns Hopkins Bloomberg School of Public Health