Finding a Better Way to Help the Sickest Kids Breathe
Clinicians and predictive analytics experts are working together to improve treatment for pediatric patients with bronchiolitis.
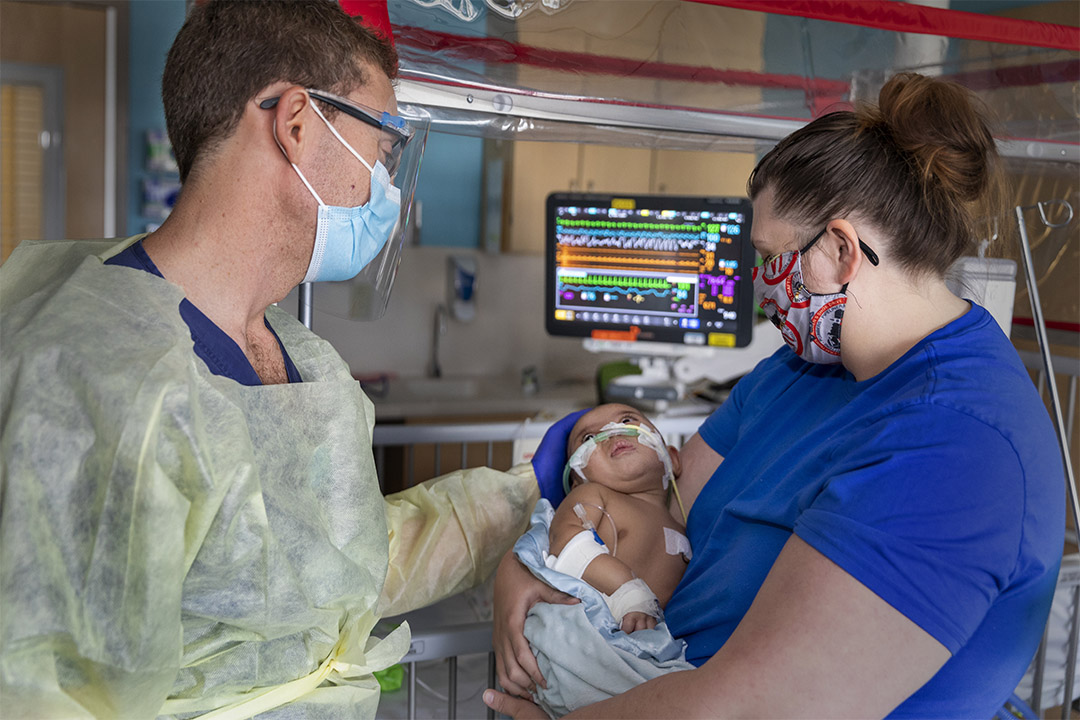
There may be nothing more frightening for parents than to see their child struggling to breathe and, after hurrying to the hospital, having their child diagnosed with bronchiolitis, a common yet serious respiratory infection among children and a leading cause for pediatric hospitalization.
The primary cause of bronchiolitis is respiratory syncytial virus (RSV), which infects the lungs and closes breathing passages. Younger children and babies are more susceptible to RSV than older children and, for them, an RSV infection can be serious.
With cases of RSV rising sharply, particularly in the southern United States, on June 10 the Centers for Disease Control and Prevention (CDC) published an RSV alert for physicians.
Severe cases of bronchiolitis require intensive respiratory care and children admitted to Johns Hopkins All Children’s with bronchiolitis may need to be treated in the pediatric intensive care unit (PICU). Children with the most serious cases of bronchiolitis may have to be intubated, which requires a tube being placed into the trachea and the child being placed on a ventilator to provide them with critical oxygen.
Intubation is very invasive and considered a “rescue therapy” of last resort. Fortunately, there are noninvasive methods for providing high levels of pressurized oxygen, and nurses and clinicians in the PICU make every effort to avoid intubating children.
Care in the PICU
Nurse Kelsey Davis, R.N., B.S.N., who has been working in Johns Hopkins All Children’s PICU for about four years, has seen many cases of severe pediatric bronchiolitis. Often, she says, most of their PICU beds are filled by children needing intensive respiratory care.
“We try our best to avoid intubation,” says Davis, recalling a particularly “close call” with an infant suffering with severe bronchiolitis and a genetic abnormality with metabolic demands that complicated treatment. “Intubation could have created developmental problems for the baby,” she says.
With careful observation and non-invasive respiratory treatment, the baby survived without being intubated.
“Her parents were happy and we were happy,” Davis recalls.
Noninvasive respiratory care
According to Anthony A. Sochet, M.D., MHSc, of the Pediatric Critical Care Medicine Division at Johns Hopkins All Children’s, little data have been gathered to identify ideal non-invasive ventilation methods used to provide respiratory care for children suffering with bronchiolitis. To assess which patients responded or did not respond to non-invasive ventilation methods, and to document how many patients were eventually intubated and why, Sochet and colleagues recently carried out several studies comparing types of nasal cannula for effectiveness.
One study compared high flow nasal cannula (HFNC) to bilevel positive airway pressure to see if there were differences in clinical outcomes in pediatric patients being treated for either severe asthma or bronchiolitis.
A second study followed 172 children admitted to the PICU with bronchiolitis and found that 33% of those children did not respond HFNC and could be predicted by following changes in the breathing rate and heart rate before and after starting HFNC.
A third study investigated the effectiveness of the Ramanathan (RAM) nasal cannula and found a 66% response rate. The 20-hour therapy mark separated responders to RAM from the non-responders, who were eventually intubated.
“Our study results provided some useful insight, which included a time course that could help clinicians identify which children are likely to be non-responders to non-invasive ventilation and which children require additional monitoring,” Sochet says. “However, we still have many questions, including how to reliably determine the timing for safely weaning a child off ventilatory support.”
With many questions unanswered, Sochet began looking for better ways to predict which children might respond to non-invasive ventilation and those who might eventually need intubation.
“Clinicians cannot be at the patient’s bedside at all times, and tests are static – they represent what is going on at a single mark in time,” Sochet says. “We need dynamic tools that provide continuous, real-time data.”
To get more dynamic tools, he knew just where to go.
Enter “predictive analytics”
Sochet has begun working with Luis Ahumada, MSCS, Ph.D., director of the Johns Hopkins All Children’s Machine Learning and Predictive Analytics Unit, to build data-driven artificial intelligence (AI) tools to predict which patients might respond to non-invasive ventilation and which may need to be intubated. They are also seeking improve outcomes for pediatric bronchiolitis patients.
The effort is part of a new frontier in medicine in which AI methods are aiding physicians in making diagnostic and treatment decisions through “clinical decision support systems” (CDSS). CDSSs use computerized algorithms — sequences of well-defined instructions that perform computations as part of data-driven “clinical pathways.”
AI is being used in many industries. The airline industry, for example, uses it to predict passenger flow. In health care, the use of AI is growing because it can provide what Ahumada calls “deep learning” by analyzing thousands of data points more quickly than can the human mind.
He explains how a “deep learning” capability of AI, enabled by algorithms, is different from simple “machine leaning.” Machine learning, a subset of AI, uses a set of algorithms to analyze and interpret structured data, learning how to evaluate similar data inputs with minimal human involvement. Deep learning, on the other hand, uses more complex algorithms in multiple layers (more than three) to create an “artificial neural network” that tries to simulate the biology of the human brain, learning from the data and making intelligent decisions by using very large datasets usually without human intervention.
AI can also capture hidden relationships in data. By observing patterns and forecasting trends captured by AI, clinicians and health care leaders can improve outcomes. Accordingly, Sochet’s objective is to develop algorithm-based AI tools dynamic enough not only to monitor patients in real-time by analyzing thousands of vital signs and clinical data points but also to predict a trajectory of care that can indicate in advance a patient’s potential for needing rescue therapy.
“AI is not a silver bullet,” Ahumada explains. “AI is not something to replace the physician. It is a tool that can assess data and analyze variables very quickly — more quickly than the human mind is able to — in real time and predict what may happen.” In this way, AI is a powerful tool in the physician’s toolbelt.
The immense amount of data that can be gathered from real time sensors constantly taking vital signs, as well as information from electronic medical records (EMRs), can be compiled to offer a “big picture” that provides what Ahumada calls “a gold mine” of information. The information “gold mine,” made available by advanced mathematical methods, powerful statistical models and machine learning algorithms, can be analyzed using predictive analytics techniques.
Research: More is better
To advance clinical research into therapeutic strategies and ultimately provide better respiratory care for children suffering from bronchiolitis, Sochet is also working with a team of collaborators based in Baltimore: Raimond Winslow, Ph.D., a professor in the Department of Biomedical Engineering and director of the Institute for Computational Medicine at Johns Hopkins University’s Homewood campus; Joseph Greenstein, Ph.D., an associate research scientist also in the Institute for Computational Medicine; and James Fackler, M.D., and Jules Bergmann, M.D., Johns Hopkins University faculty-physicians in pediatric critical care at Johns Hopkins Children’s Center.
Sochet and his colleagues are mentoring a team of biomedical engineering students in a course called “Precision Care Medicine” to address these cutting-edge research questions. The team has developed and evaluated several novel machine learning models and compared their performance to existing models, such as the respiratory rate oxygenation (ROX) index, which has been validated to predict HFNC outcomes in patients with pneumonia. It has not been clear whether the ROX index would perform as well in patients with respiratory failure from causes other than pneumonia, such as pediatric patients with acute bronchiolitis.
In their retrospective cohort study of children receiving HFNC for acute respiratory failure, “tree-based” machine learning models developed by the team outperformed the ROX index in predicting future flow rate escalations in pediatric bronchiolitis patients.
“This is an example of applying near real-time data, high-resolution data to improve the practice of medicine. The goal is to allow computers to assist in identifying patients who may deteriorate and potentially modifiable factors well in advance. We believe we can predict who will possibly fail therapy and can find alternate solutions with time to spare,” Sochet concludes.
Through the efforts of Johns Hopkins All Children’s Machine Learning and Predictive Analytics Unit and biomedical engineering research applied in the PICU to help the sickest children breathe, the future of pediatric bronchiolitis care will likely be brighter, as well as more predictable.