Researchers at Johns Hopkins All Children's Take Major Step Forward in Harnessing Data for Future Advances in Patient Care
A project at the hospital is making better-quality pediatric data more available to researchers throughout the Johns Hopkins Health System.
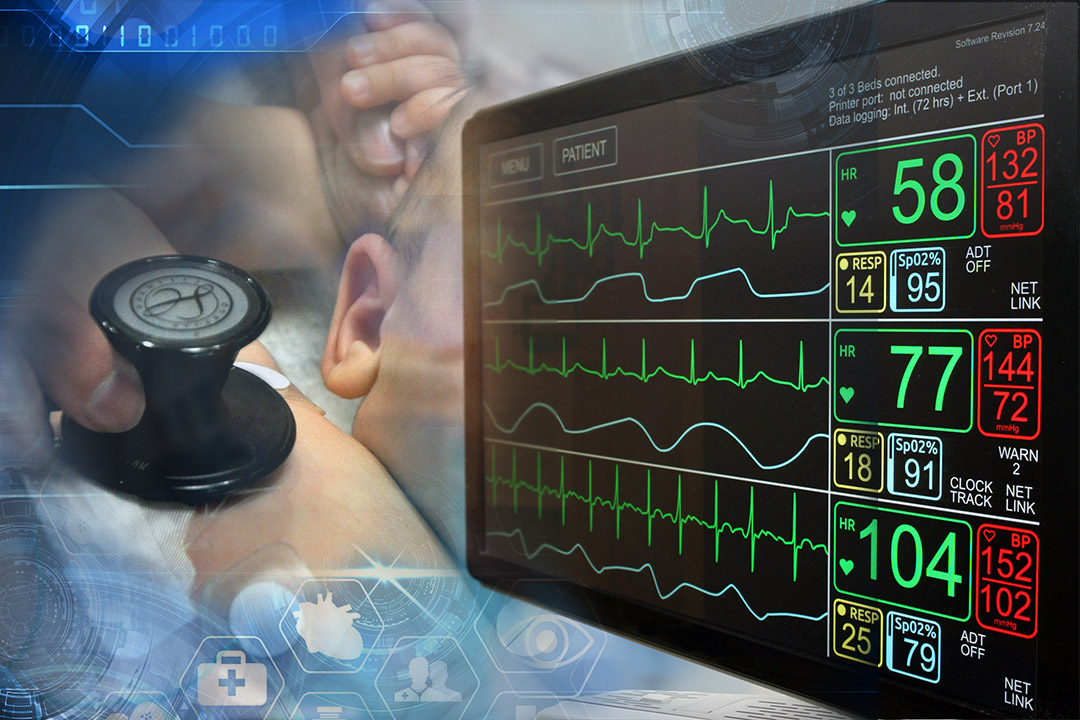
Johns Hopkins All Children’s Hospital has advanced the way it collects data for research purposes in some areas of the hospital, which will impact research related to children’s health.
Researchers at Johns Hopkins All Children’s Hospital envision a day when they can predict which patients are most likely to experience an event such as breathing problems under anesthesia, for example, and prevent it before it happens.
It’s a future that’s getting closer and closer as researchers and data scientists at the St. Petersburg, Florida, hospital take an important step in how they harness the data that will make this kind of lifesaving prevention an eventual real-time reality.
Johns Hopkins All Children’s is now one of several children’s hospitals that collects continuous vital sign data from patients in critical care areas of the hospital, making better-quality pediatric data more available to researchers throughout the Johns Hopkins Health System. Rather than only retaining the data for a year or so, using cloud technology the team at the hospital is now able to essentially save the information permanently, allowing researchers to have access to larger sample sizes of better-quality data.
The data collected for research purposes are “de-identified,” which means they are not tied to individual patients, so all patients’ privacy is protected. Like other research studies involving data collected from patients, the study was reviewed and approved by the Johns Hopkins All Children’s Institutional Review Board.
“We can derive rules and provide recommendations using machine learning to identify potential events and diagnoses in hospitalized children before they happen. That’s the holy grail,” says Luis Ahumada, Ph.D., director of predictive analytics at Johns Hopkins All Children’s. It’s a process that has only just begun. Next steps would include further in-depth research involving not only Johns Hopkins All Children’s but also other institutions to validate data, and later robust clinical trials.
“Once we develop these algorithms using the high-resolution data we’re now collecting, we’re going to create novel predictive models using artificial intelligence that will help us identify certain medical conditions or events ahead of time,” says Ali Jalali, Ph.D., data scientist at Johns Hopkins All Children’s.
The first part of the project involved updating the monitors in the operating rooms and the Center for Congenital Diaphragmatic Hernia (CDH) to ones that could communicate with both the electronic medical record that doctors and nurses use in daily patient care, as well as the cloud-based storage system the hospital uses to retain de-identified data for research.
The rest of the patient care areas in the hospital’s intensive care units also received this upgrade in recent months. Intensive care unit beds account for 57% of the hospital’s beds. The CDH Center represents an additional 15 beds in a unit where children receive advanced, highly specialized care.
The hospital is working with the Baltimore-based Johns Hopkins Precision Medicine Analytics Platform team to store and begin analyzing data.
The hospital’s new monitors can potentially collect nearly 1,000 continuous data inputs on vital signs from a single patient – everything from blood pressure to variations in a patient’s heart rate. Combined with demographic data like age, medications being administered, and a child’s medical conditions – it would be nearly impossible for humans to “process” that information, compare it against information gathered from other patients and predict outcomes in real time. That’s where machine learning comes in.
Machine learning involves giving a computer the capability to receive information and learn and improve on its knowledge of the topic without being further programmed. With the new computing power, researchers are developing algorithms that ultimately could help to identify patients who might be at risk for an event like breathing issues under anesthesia before it happens. For instance, the computer might identify patterns among thousands of children whose medical condition acutely worsened and recognize similarities in the data before this happens in other patients, alerting doctors to consider an intervention.
“With this data capture, we can more easily look at things like beat-to-beat variability, or variations in pulse oximetry (oxygen levels in the blood), for example,” says Mohamed Rehman, M.D., Chair of the Department of Anesthesia and Pain Medicine and Director of Perioperative Health Informatics. “We are moving from a very high level of data to a very granular level of data.”
One of the areas that the team is focusing on is laryngospasm, which is a sudden closure of the vocal cords that temporarily makes it difficult to breathe or speak. In people who are awake and fully conscious, it most often occurs when someone is choking on food, for example, and it is a reflex meant to prevent anything other than air from entering the lungs.
When a patient is under anesthesia, the reflex can inadvertently occur, and this can be life threatening if not recognized quickly by the anesthesiologist.
Physicians and researchers have accumulated knowledge about laryngospasm – they know, for example, that it is more common in younger children and it is more likely to occur right after a patient has received anesthesia and has fallen asleep, or just as they are coming out of anesthesia. Also, it is seen more frequently in surgeries involving the throat, mouth or trachea (wind pipe) as opposed to surgery on the ankle, for example.
But the team wants to dive deeper. The potential with machine learning comes in being able to take huge amounts of data from one patient, and cross reference it with data from other patients who experienced the same event, and pinpoint potential risk factors or combinations of risk factors. If a computer can identify these risk factors in real time, and alert physicians that their patient meets a certain number of criteria, this can help the physicians prevent an issue before it occurs.
Much work will be done before this sort of machine learning will be used in patient care, but this advance in data collection lays the foundation.
“You cannot do research unless you collect data so we have set up the infrastructure for data capture and storage, and integrate the data with several other data sets. Now this forms a very good infrastructure to go to the next level,” Rehman says. “We’ve formed the foundation for new wisdom. New wisdom comes from new knowledge, and new knowledge comes from better data.”